What is generative AI? Everything you need to know
Any idea what the ‘G’ in ChatGPT stands for? Full marks if you answered ‘Generative’.
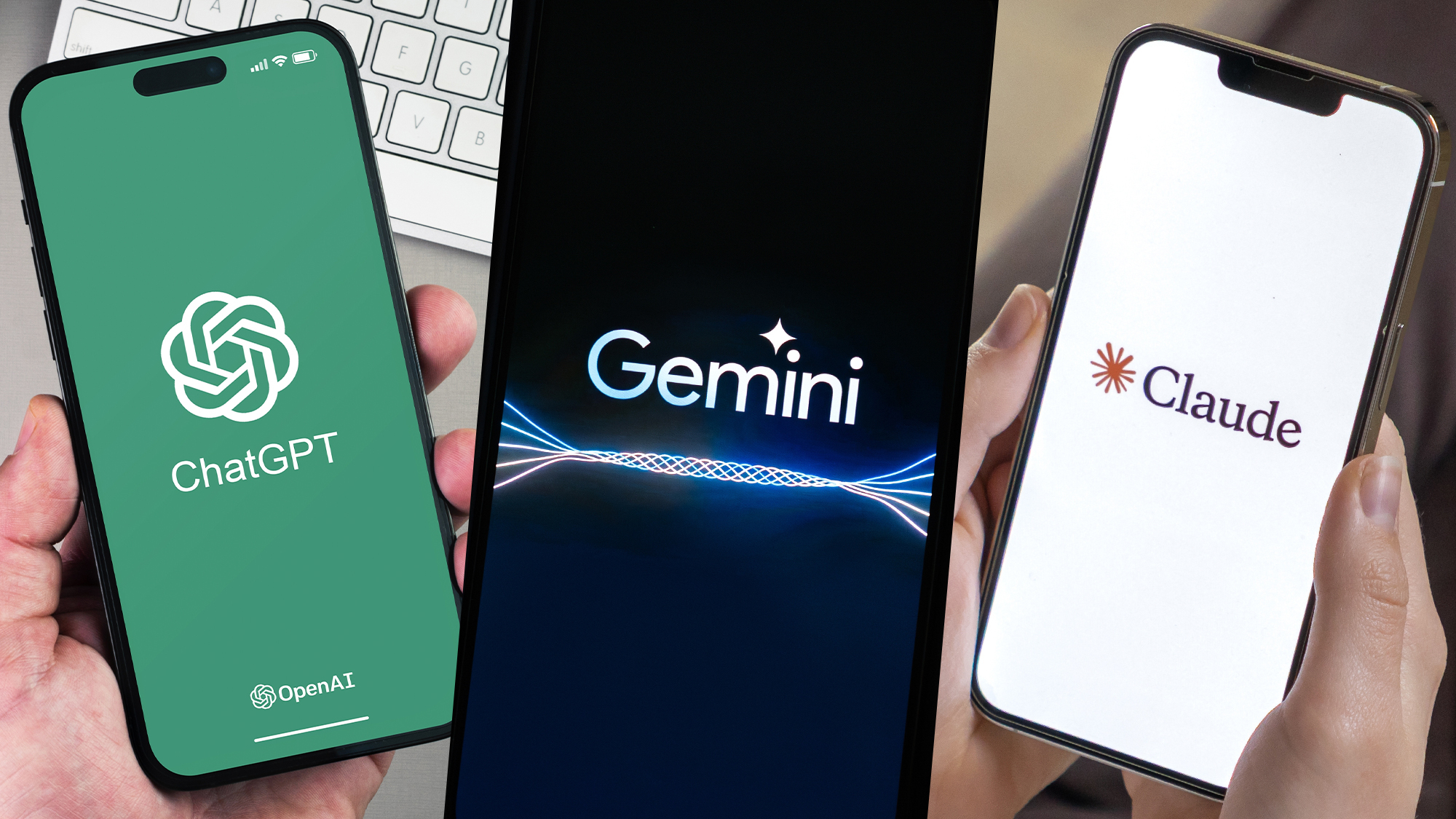
OpenAI’s flagship artificial intelligence chatbot — along with the best ChatGPT alternatives like Google Gemini, Microsoft Copilot and Anthropic’s Claude — are all examples of generative AI models.
Using generative AI technology has become an integral part of many people’s personal and professional lives. But what does generative AI (often abbreviated to GenAI) actually mean, what distinguishes it from other types of artificial intelligence, and how does it work? You can find answers to all those questions below — assuming you haven’t already asked ChatGPT, of course.
What is generative AI?
At the risk of jeopardizing my journalists’ guild card, it seems appropriate in this instance to throw over to ChatGPT for a definition of generative AI:
“Generative AI is a type of artificial intelligence that creates new content—such as text, images, music, or code—by learning patterns from existing data. It uses models like GANs and transformers to produce realistic, human-like outputs, enabling creative applications in art, design, writing, and other fields.”
Or, put even more briefly: artificial intelligence that generates content.
Although usage of the expression ‘generative AI’ is relatively recent, the concept has been around for around three quarters of a century — computer scientist Arthur Samuel popularized the term ‘machine learning’ in the 1950s, which can be seen as a forebear for generative AI.
While research and progress was made over the decades, generative AI as we know it made its biggest strides only a decade ago, thanks to the development of Generative Adversarial Networks (GANs, as referred to in the definition above) by engineer Ian Goodfellow.
Get instant access to breaking news, the hottest reviews, great deals and helpful tips.
This was closely followed in 2017 by the introduction by scientists at Google of ‘transformer architecture’, which is the base for the generative AI tools that are most commonly used today.
What are some examples of generative AI?
If you’ve used a popular chatbot tool like ChatGPT, Gemini, Copilot or Claude, then you’ve used generative AI. So that’s anytime you’ve asked it for restaurant recommendations, help with an essay, or a template letter to complain to your landlord.
Its uses range from harmless fun (devising original poems and songs or fantastical images), to professional (creating presentations, designing product prototypes, strategizing), and all the way to potentially lifesaving (drug discovery).
Many social media trends — such as visualizing your very own action figure or turning your dog into a human — are a product of generative AI.
However, generative AI has also been utilized for more nefarious means. Deepfakes used to spread misinformation, damage people’s reputations or create ‘nude’ images for sextortion scams, are one reason why the proliferation of generative AI worries so many people; especially as the technology becomes ever more convincing and easy to use.
How does generative AI work?
Don’t worry — I’m not going to explore the depths of probabilistic modeling and high-dimensional outputs here. In fact, in very simple terms, you can think of generative AI models carrying out two core functions.
Their first job is to learn patterns from massive sets of data. These datasets include text, images, web pages, code, and anything else that can be fed into the model; this is commonly known as ‘training’.
The AI model then identifies patterns in that data, effectively acquiring knowledge and understanding techniques. For example, if the model was fed the 100 greatest horror novels ever written, it would cross-reference the data to draw out the structure, language, themes, and narrative devices common to those books.
Next, it applies that training to generate something completely new. So when you ask ChatGPT to plan your next vacation, it takes all the information it has gathered and uses something called ‘learned probability distribution’ to compose the response.
Where this is a written response, it does this by working on a word-by-word basis, using its acquired data to select the most appropriate next word of the sentence. Or for images, generative AI tools using transformer-based models take in the colours and composition of the myriad real images it’s seen. Ask Midjourney to create a comic strip, for example, and it is likely considering all of the samples it has previously been trained on to produce something that accurately fits the brief.
These two terms are often used interchangeably, which can be a bit confusing. AI is an umbrella term to cover all forms of artificial intelligence. Generative AI sits under that umbrella, but refers specifically to AI tools that generate content.
A notable illustration of the difference is IBM’s chess-playing computer Deep Blue, which famously defeated Garry Kasparov — one of history’s greatest human chess players — in 1997. The computer was built using so-called symbolic AI to learn moves, evaluate games and make strategic decisions, but wouldn’t be classed as generative AI as it does not create anything new.
Another common example of non-generative AI is discriminative AI. This is used in the facial recognition software that groups photos together in your smartphone’s photo album or that discerns spam emails and hides them from your inbox.
So while chatbots like ChatGPT, Copilot and Gemini certainly come under the big AI umbrella, they’re more accurately categorized as generative AI models.
Challenges of generative AI
While we touched on the malicious use of generative AI above, other drawbacks of generative AI are more an integral product of the way the tech works. These models are only ever as good as the information that they’re trained on. Believe it or not, there’s quite a lot of outdated, misleading or plain wrong information on the internet — all of which can be pulled into a chatbot’s orbit and then regurgitated out as fact. These errors can also be known as .’hallucinations’.
For the same reason, generative AI models can also fall into the trap of reaffirming biases or stereotypes. As per an example given by ChatGPT itself: “Text-to-image models often associate professions like ‘nurse’ with women and ‘CEO’ with men.”
Academic institutions have been pulling their hair out in trying to deal with students using ChatGPT and the like to write essays and dissertations. While the challenges it poses to creative industries — could generative AI really make writers, actors, musicians and artists entirely superfluous? — is a perpetual source of debate.
More from Tom's Guide
- This tiny prompt change makes ChatGPT way more useful — here’s how
- How to make and run your own AI models for free
- Apple agrees to $95 million Siri settlement — here’s how to claim your share












Adam was the Content Director of Subscriptions and Services at Future, meaning that he oversaw many of the articles the publisher produces about antivirus software, VPN, TV streaming, broadband and mobile phone contracts - from buying guides and deals news, to industry interest pieces and reviews. Adam can still be seen dusting his keyboard off to write articles for the likes of TechRadar, T3 and Tom's Guide, having started his career at consumer champions Which?.
You must confirm your public display name before commenting
Please logout and then login again, you will then be prompted to enter your display name.