How 'Anonymous' Shopping Data Reveals Your Identity
Scrubbed credit-card purchase data can easily be used to track individuals, and Big Data may never truly be anonymous, a new study finds.
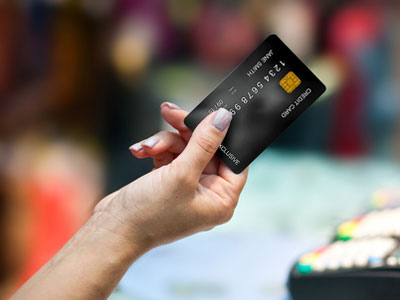
Your personal shopping habits can be used to identify you with 90 percent accuracy — and the trackers don't need your name, address or even credit-card number, a new study finds.
Women are easier to identify than men, rich people are easier to identify than poorer ones, and, given a large enough data set, true anonymity may be mathematically impossible, according to the study, published in the Jan. 30 issue of the journal Science. The findings may require re-examination of the entire practice of gathering "big data."
MORE: 13 Security and Privacy Tips for the Truly Paranoid
Even many people who aren't tech-savvy know that breadcrumbs of data can be used to track an individual's movements, provided that the tracker is armed with a name or some other kind of personally identifiable information (PII).
Yves-Alexandre deMontjoye, a graduate student at the Massachusetts Institute of Technology, looked at the metadata from credit-card records — not what was bought and who bought it, but instead the time, date, place and price of each transaction. Cardholder names and any other obvious identifiers were scrubbed out, and card account numbers were replaced by randomly assigned ID numbers.
In 90 percent of the cases, it was possible to link those random ID numbers to individuals from only four pieces of metadata — and sometimes only three, since the time of day wasn't always necessary, de Montjoye told Tom's Guide.
"We were really trying to quantify how many pieces of information were needed," de Montjoye said.
Sign up to get the BEST of Tom's Guide direct to your inbox.
Get instant access to breaking news, the hottest reviews, great deals and helpful tips.
The credit-card data was provided by a single bank in an unnamed country and covered the three months from Jan. 1, 2014 to March 31, 2014, yielding data from 1.1 million cards used in 10,000 shops. DeMontjoye wouldn't name the country involved, but said it was one of the 34 members of the Organization for Economic Cooperation and Development — a rich, probably Western, country.
Putting a face to a number
The reason for the accuracy of identification is actually pretty simple. For example, Jane Doe — whom the researcher would know as only an alphanumerical ID such as "7abc123a" — might be one of 1,000 people to use a credit card in a certain pizza shop on a given day. But there would be far fewer people who would use credit cards at both that pizza shop and a certain shoe store on that day, and fewer still who would buy things at three different specific shops on the same day.
From that point, it would be possible to track down other places to which Jane Doe had gone by combing through the database of 1.1 million cards to pull out all her activity. Tack on the price of each transaction — even a price range will do — and the odds of linking Jane to a real name go up dramatically. In fact, with just a few data points, you'd be able to identify an individual user roughly 90 percent of the time, de Montjoye found.
Say Ms. Doe begins each weekday by buying coffee at a Starbucks near Union Square in Manhattan. She often buys lunch at any of half a dozen markets and takeout restaurants nearby. But she buys a subway MetroCard in Park Slope, Brooklyn, and used her credit card at a drycleaner's in the same neighborhood.
We've established roughly where Jane Doe lives and works. But she also buys clothes at the upscale department store Barneys, and often uses the online taxi service Uber to get around New York on nights and weekends. Now we know that she makes a comfortable income.
Collect such data over three months, build up a profile, and then correlate it with publicly available information — such as personal profiles on LinkedIn or Facebook, or where people "check in" on Foursquare — about individuals who fit that profile, and you'll probably be able to match the randomized ID with Jane Doe.
The method is even more useful if you already know who you are seeking. Say you're the FBI and you want to track Jane Doe, but only have a name and address and a stack of anonymous credit card data. Montjoye's method makes it simple to match the two up.
"We also studied the effects of gender and income on the likelihood of re-identification," de Montjoye wrote in the paper. "The higher somebody's income is, the easier it is to re-identify him or her. … The odds of women being re-identified are 1.214 times greater than for men."
Crunching data to spit out names
This isn't the first time anyone has studied re-identification of individuals. In 2006, America Online released a database of the search queries of 650,000 AOL users, and researchers quickly found out how to match them up with names using publicly available information. They were able to do so because the anonymization consisted merely of replacing the names with a unique identifier.
The same year, Netflix published a trove of movie recommendations and asked for help from the public in coming up with a better algorithm. But Arvind Narayanan and Vitaly Shmatikov, researchers at the University of Texas at Austin, were able to reconstruct the names attached to them by comparing the data to public information on the Internet Movie Database (imdb.com) — in that case, recommendations from users.
De Montjoye's study takes those methods one step further. It shows that even within a database, it's possible to entirely remove personally identifiable information and still end up with unique identifiers. Only a few data points are needed, and, from there, it's no great feat to merge it with another data set.
More to the point, typical methods of anonymization probably won't work, de Montjoye said. The implication is that given a large enough data set, true anonymization of data might be a mathematical impossibility.
The implication is that "Big Data" can never truly be anonymized. Given enough data — but far less than what’s available to Google, Facebook, Amazon, Apple or Microsoft, not to mention a marketing-research company such as Acxiom— it's almost certain that a data set can be matched to a real name.
The findings don't surprise Susan Landau, a professor of cybersecurity policy at Worcester Polytechnic Institute in Massachusetts.
"I use an anonymous travel card," Landau said. "The travel we do as tourists — if you know the area of the hotel, combined with the day, you could figure out who we were."
Can you limit deanonymization?
For organizations such as the National Security Agency or Facebook, the deanonymization provided by large data sets is a feature, not a bug. The NSA wants to see as much data about as many individuals as possible, in the name of security, and a big piece of Facebook's business model is selling ads tailored to the interests of users. There are also legitimate reasons to gather large amounts of data such as these for medical and population studies.
As long as the data is going to be collected, Landau said, the key to privacy is to control the data's use and the information derived from it. She noted that, in the medical-research community, scientists who leak personal data can be denied access to the data sets for a time. In that case, the people who use the data self-police.
"If you can't get the data, you're done as a researcher," Landau said.
Lee Tien, senior staff attorney at the Electronic Frontier Foundation, a digital-rights and privacy advocacy group in San Francisco, said such problems should prompt system designers to rethink how data is gathered. Rather than picking up as much information as possible, Tien said, it might be better to think through exactly what's needed and, most importantly, not keep it around for long.
"One way to do this." Tien said, "is to say [that] entities should not pick it up unless it's absolutely necessary."
MORE: Can You Hide Anything from the NSA?
It's also possible to offer data that has the same statistical relationships as the data one wants to study, but to pepper it with "false" information in the fields that aren't relevant, Tien said. He noted that the U.S. Census Bureau does this when giving out data to researchers.
De Montjoye added that his research really suggests that the concept of personal data should be rethought. The French agency that governs data privacy, the Commission nationale de l'informatique et des libertés, approaches private data by asking that data sets be "provably anonymous."
"That doesn't scale," de Montjoye said, "and it's probably not achievable."
De Montjoye said his findings don't indicate that the practice of gathering data is bad itself, but rather that it might be necessary to come up with a better notion of what kinds of data are truly personal.
Data gathering now "relies on this vague notion of personal data, either defined as names or PII," he said. "We're showing this is not enough."
- 10 Facebook Privacy and Security Settings to Lock Down
- How to Be Anonymous Online
- 7 Ways to Lock Down Your Online Privacy
Follow us @tomsguide, on Facebook and on Google+.